Exploring Daron Acemoglu’s Institutional Theory: Integrating Machine Learning, Human Behavior & Quantum Computation in Financial Modeling
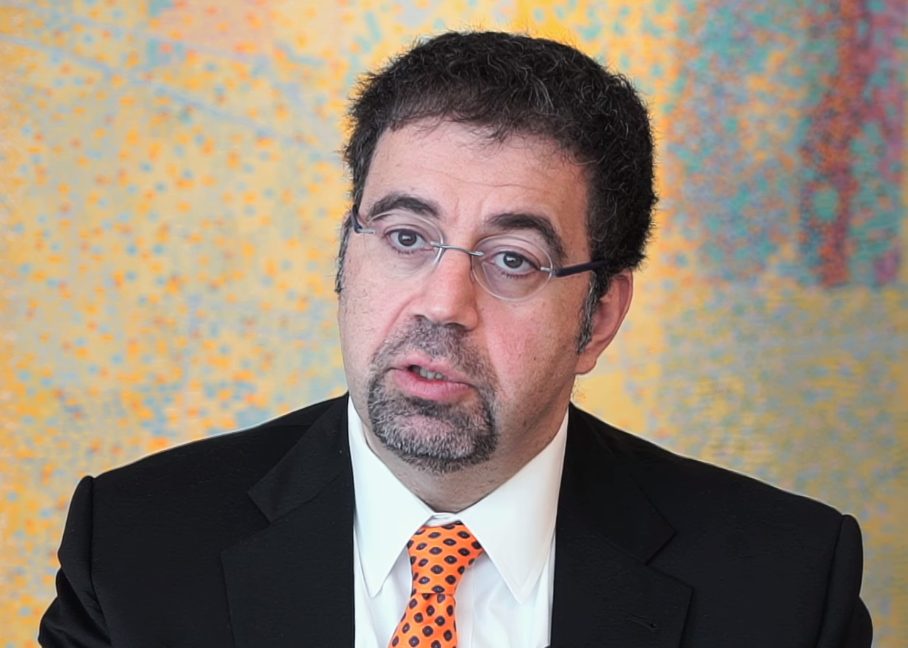
Abstract
Daron Acemoglu’s extensive work on institutional economics has reshaped our understanding of economic development by emphasizing the pivotal role of political and economic institutions. However, critiques suggest that his theories may overlook other critical factors such as geography, culture, and technology. This article delves deep into Acemoglu’s perspectives, examines counterarguments, and explores the complexities of predictive modeling in finance. We highlight the significance of accurate parameter selection and correlation analysis in machine learning models, discuss the challenges posed by the stochastic nature of human behavior in financial markets, and propose quantum computation as a potential solution to these challenges.
Introduction
The quest to understand why nations experience divergent economic trajectories has long captivated economists, historians, and policymakers. Daron Acemoglu, a leading economist and professor at the Massachusetts Institute of Technology (MIT), has contributed significantly to this discourse through his institutional theory of economic development. His work, particularly in collaboration with political scientist James A. Robinson, posits that inclusive institutions are the fundamental drivers of prosperity.
While Acemoglu’s theories have gained widespread recognition, they are not without criticism. Some scholars argue that his emphasis on institutions neglects other vital factors like geography, culture, and technological innovation. Furthermore, as we advance into an era where machine learning and artificial intelligence are increasingly employed in economic and financial modeling, new challenges arise—particularly in accounting for the randomness and complexity of human behavior.
This article aims to:
- Provide an in-depth analysis of Daron Acemoglu’s work and perspectives.
- Explore counterarguments that highlight overlooked factors in his theories.
- Discuss the importance of accurate parameter selection and correlation analysis in machine learning.
- Examine the difficulties of incorporating human nature into financial models.
- Propose quantum computation as a potential solution to these challenges.
Daron Acemoglu’s Institutional Theory
Foundations of Institutional Economics
At the core of Acemoglu’s work is the assertion that institutions—defined as the formal and informal rules governing economic, political, and social interactions—are the primary determinants of economic outcomes. Institutions shape incentives, influence behavior, and ultimately determine the allocation of resources within a society.
Inclusive vs. Extractive Institutions
Acemoglu and Robinson categorize institutions into two types:
- Inclusive Institutions: These institutions allow and encourage participation by the masses in economic activities. They feature secure property rights, unbiased legal systems, and public services that provide a level playing field. Inclusive political institutions are pluralistic and distribute power broadly, preventing any single group from monopolizing control.
- Extractive Institutions: In contrast, extractive institutions are designed to extract wealth and resources from one subset of society to benefit another. They concentrate power in the hands of a few, lack property rights protections, and often suppress economic progress to maintain the status quo.
Historical Evidence
In Why Nations Fail, Acemoglu and Robinson employ a historical approach to support their thesis. They examine cases like the divergent paths of North and South Korea, the impact of the Glorious Revolution in England, and the economic stagnation in many African nations post-colonization. These examples illustrate how institutional differences, rather than geographic or cultural factors, have led to varying economic outcomes.
The Role of Critical Junctures and Path Dependence
Acemoglu emphasizes the importance of critical junctures—significant events that disrupt existing institutions and allow for new ones to emerge. The concept of path dependence suggests that once a society embarks on a particular institutional path, it tends to continue along it due to increasing returns and vested interests.
Mathematical Modeling of Institutions
Acemoglu employs game theory and mathematical models to formalize his theories. For instance, he uses dynamic stochastic general equilibrium (DSGE) models to analyze how institutions affect economic variables like investment, innovation, and growth. These models incorporate rational agents making decisions based on their expectations of future policies and economic conditions.
Counterarguments and Overlooked Factors
While Acemoglu’s institutional theory is influential, several scholars argue that it may oversimplify or neglect other crucial determinants of economic development.
Geography and Ecology
Jeffrey Sachs’ Geographical Hypothesis
Economist Jeffrey Sachs contends that geography plays a significant role in economic development. Factors such as climate, topography, and access to the sea can influence agricultural productivity, disease prevalence, and transportation costs.
- Climate Impact on Agriculture: Tropical climates often have less fertile soils and are prone to diseases affecting both crops and livestock, hindering agricultural output.
- Disease Burden: Higher incidences of diseases like malaria can reduce labor productivity and increase healthcare costs.
- Resource Distribution: The presence or absence of natural resources can shape economic opportunities and constraints.
Empirical Evidence
Studies using cross-country regression analyses have found correlations between geographical variables and economic performance. For example, landlocked countries often have lower GDP per capita due to higher transportation costs affecting trade.
Cultural Influences
Max Weber’s Protestant Ethic Thesis
Sociologist Max Weber argued that cultural values, particularly those stemming from Protestantism, fostered attitudes conducive to economic success—such as hard work, frugality, and efficiency.
Modern Cultural Theories
Contemporary researchers examine how trust, social capital, and cultural norms influence economic transactions and institutional effectiveness. High-trust societies may experience lower transaction costs and more robust economic interactions.
Technological Innovation
Endogenous Growth Theory
Economists like Paul Romer emphasize the role of technological innovation as an engine of economic growth. Innovation leads to new products and processes, increasing productivity and creating positive externalities.
Technology Diffusion
The spread of technology across borders can enhance growth even in countries with less favorable institutions, suggesting that technology can independently impact economic outcomes.
Globalization and External Forces
International Trade and Investment
Global market dynamics, such as trade liberalization and foreign direct investment (FDI), can significantly influence domestic economies. Access to global markets allows countries to specialize and benefit from comparative advantages.
Dependency Theory
Some scholars argue that global economic structures can perpetuate inequality, where developed nations benefit at the expense of developing ones through unfavorable trade terms and capital flows.
The Complexity of Financial Modeling
Importance of Accurate Parameter Selection
In financial modeling, especially using machine learning (ML), the selection of parameters—or features—is critical for predictive accuracy.
Feature Engineering
- Relevance: Parameters must be relevant to the target variable. Irrelevant features can introduce noise and reduce model performance.
- Redundancy: Highly correlated features can lead to multicollinearity, complicating the interpretation of model coefficients.
- Dimensionality Reduction: Techniques like Principal Component Analysis (PCA) help reduce the number of parameters while retaining essential information.
Mathematical Underpinnings
- Covariance and Correlation Matrices: Understanding the relationships between variables is crucial. The covariance matrix Σ\SigmaΣ represents the pairwise covariances between variables, which is essential in multivariate analyses.
- Regularization Techniques: Methods like Lasso and Ridge regression introduce penalties for complexity, helping prevent overfitting by discouraging the model from fitting the noise.
Understanding Parameter Correlations
Multicollinearity Issues
When independent variables are highly correlated, it becomes challenging to isolate their individual effects on the dependent variable. This can inflate the variance of coefficient estimates and make the model unstable.
Variance Inflation Factor (VIF)
The VIF quantifies the extent of multicollinearity. A VIF greater than 10 indicates significant multicollinearity, suggesting the need for corrective measures.
Causal Inference vs. Prediction
While correlation does not imply causation, in predictive modeling, the focus is often on the ability to predict rather than explain. However, understanding causality can improve model robustness and interpretability.
Challenges in Modeling Human Behavior in Finance
Behavioral Economics
Traditional financial models assume rational agents maximizing utility. However, behavioral economics reveals that individuals often deviate from rationality due to cognitive biases and emotions.
Common Cognitive Biases
- Overconfidence Bias: Investors overestimate their knowledge and ability to predict market movements.
- Herd Behavior: Individuals may follow the crowd, leading to asset bubbles or crashes.
- Loss Aversion: The pain of losses is felt more intensely than the pleasure of gains, influencing risk-taking behavior.
Randomness and Stochastic Processes
Financial markets exhibit stochastic behavior, with price movements often modeled as random walks or geometric Brownian motion. However, real markets display properties like volatility clustering and fat tails, challenging these assumptions.
Mathematical Models Incorporating Human Behavior
- Agent-Based Models (ABM): Simulate interactions of autonomous agents to assess their effects on the system. ABMs can incorporate heterogeneous agents with varying behaviors.
- Stochastic Differential Equations (SDEs): Model the dynamics of asset prices with random components. The inclusion of jump processes can account for sudden market movements.
Limitations of Traditional Machine Learning Models
Overfitting to Historical Data
Machine learning models trained on historical data may not generalize well to future unseen data, especially if underlying patterns change due to shifts in human behavior.
Non-Stationarity
Financial time series are often non-stationary, meaning their statistical properties change over time. This violates the assumptions of many ML algorithms, leading to unreliable predictions.
Curse of Dimensionality
As the number of parameters increases, the volume of the feature space grows exponentially, requiring exponentially more data to achieve the same level of accuracy.
Quantum Computation as a Potential Solution
Overview of Quantum Computation
Quantum computers leverage the principles of quantum mechanics, such as superposition and entanglement, to perform computations fundamentally differently from classical computers.
Quantum Bits (Qubits)
Unlike classical bits, which are either 0 or 1, qubits can exist in a superposition of states, allowing quantum computers to process a vast amount of possibilities simultaneously.
Quantum Algorithms for Finance
Quantum Machine Learning (QML)
Quantum algorithms can enhance machine learning through:
- Quantum Support Vector Machines: Utilize quantum kernels to handle high-dimensional data more efficiently.
- Quantum Neural Networks: Leverage quantum circuits to model complex patterns that classical neural networks may struggle with.
Solving Optimization Problems
Many financial problems, such as portfolio optimization, are NP-hard. Quantum algorithms like Grover’s algorithm can search unsorted databases quadratically faster, and quantum annealing can find global minima more efficiently.
Modeling Complex Correlations
Quantum computers can naturally handle complex, high-dimensional probability distributions due to their ability to exist in multiple states simultaneously.
Handling Non-Stationary and Stochastic Processes
Quantum algorithms can be designed to adapt to changing patterns, potentially offering better modeling of non-stationary time series.
Mathematical Foundations
- Quantum Fourier Transform (QFT): Provides exponential speedups in certain computational tasks, useful in signal processing and time series analysis.
- Quantum Amplitude Amplification: Enhances the probability of desired outcomes, which can improve sampling methods in Monte Carlo simulations.
Challenges and Limitations
- Decoherence: Quantum states are fragile and can be disrupted by environmental interactions.
- Scalability: Building quantum computers with a large number of qubits remains a technological challenge.
- Algorithm Development: Quantum algorithms are still in their infancy, and developing new algorithms requires expertise in both quantum mechanics and computational complexity.
Integrating Quantum Computation into Financial Modeling
Potential Applications
- Risk Analysis: Quantum computers can process complex risk models that incorporate a multitude of variables and their interdependencies.
- Market Simulation: Simulating entire markets with numerous interacting agents may become feasible, providing deeper insights into potential market behaviors.
- Real-Time Data Processing: Quantum computation could enable the processing of massive data streams in real-time, allowing for instantaneous adjustments to models based on the latest information.
Case Studies and Research
- Quantum Monte Carlo Methods: Used for option pricing and risk assessment, potentially offering speedups over classical Monte Carlo simulations.
- Quantum Portfolio Optimization: Early experiments demonstrate the ability to optimize portfolios more efficiently, taking into account a broader set of constraints and objectives.
Interdisciplinary Collaboration
Advancements in this area require collaboration across multiple disciplines:
- Economists and Financial Analysts: Provide domain knowledge and identify relevant problems.
- Physicists and Mathematicians: Develop the theoretical frameworks and algorithms.
- Computer Scientists and Engineers: Build the hardware and software infrastructure.
Conclusion
Daron Acemoglu’s focus on institutions as the primary drivers of economic development has significantly influenced economic thought. However, the complexities of real-world economies necessitate a broader perspective that includes geography, culture, technology, and globalization. In the realm of finance, modeling these complexities is further challenged by the unpredictable nature of human behavior.
Machine learning offers powerful tools for predictive modeling but faces limitations due to the randomness and non-stationarity inherent in financial markets. Quantum computation emerges as a promising solution, potentially revolutionizing our ability to model and predict complex systems by handling vast amounts of data and intricate correlations more efficiently.
While quantum computing is still developing, its integration into financial modeling could lead to more accurate predictions, better risk management, and a deeper understanding of market dynamics. Embracing interdisciplinary approaches and continuing to explore these advanced computational methods will be essential in addressing the challenges posed by the ever-evolving landscape of global finance.
References
- Acemoglu, D., & Robinson, J. A. (2012). Why Nations Fail: The Origins of Power, Prosperity, and Poverty. Crown Publishers.
- Sachs, J. D. (2001). “Tropical Underdevelopment.” National Bureau of Economic Research Working Paper No. 8119.
- Weber, M. (1905). The Protestant Ethic and the Spirit of Capitalism. Routledge Classics.
- Romer, P. M. (1990). “Endogenous Technological Change.” Journal of Political Economy, 98(5), S71-S102.
- Fama, E. F. (1970). “Efficient Capital Markets: A Review of Theory and Empirical Work.” The Journal of Finance, 25(2), 383-417.
- Kahneman, D., & Tversky, A. (1979). “Prospect Theory: An Analysis of Decision under Risk.” Econometrica, 47(2), 263-291.
- Farmer, J. D., & Foley, D. (2009). “The Economy Needs Agent-Based Modelling.” Nature, 460(7256), 685-686.
- Biamonte, J., Wittek, P., Pancotti, N., Rebentrost, P., Wiebe, N., & Lloyd, S. (2017). “Quantum Machine Learning.” Nature, 549(7671), 195-202.
- Rebentrost, P., Schuld, M., Wossnig, L., Petruccione, F., & Lloyd, S. (2018). “Quantum Gradient Descent and Newton’s Method for Constrained Polynomial Optimization.” New Journal of Physics, 21(7), 073023.
- Orús, R., Mugel, S., & Lizaso, E. (2019). “Quantum Computing for Finance: Overview and Prospects.” Reviews in Physics, 4, 100028.
- Markowitz, H. (1952). “Portfolio Selection.” The Journal of Finance, 7(1), 77-91.
- Shor, P. W. (1994). “Algorithms for Quantum Computation: Discrete Logarithms and Factoring.” Proceedings 35th Annual Symposium on Foundations of Computer Science, 124-134.
- Nielsen, M. A., & Chuang, I. L. (2010). Quantum Computation and Quantum Information. Cambridge University Press.
- Sutton, R. S., & Barto, A. G. (2018). Reinforcement Learning: An Introduction. MIT Press.
- Grover, L. K. (1996). “A Fast Quantum Mechanical Algorithm for Database Search.” Proceedings of the Twenty-Eighth Annual ACM Symposium on Theory of Computing, 212-219.