New AI Tool Accurately Predicts Colon Cancer Treatment Response
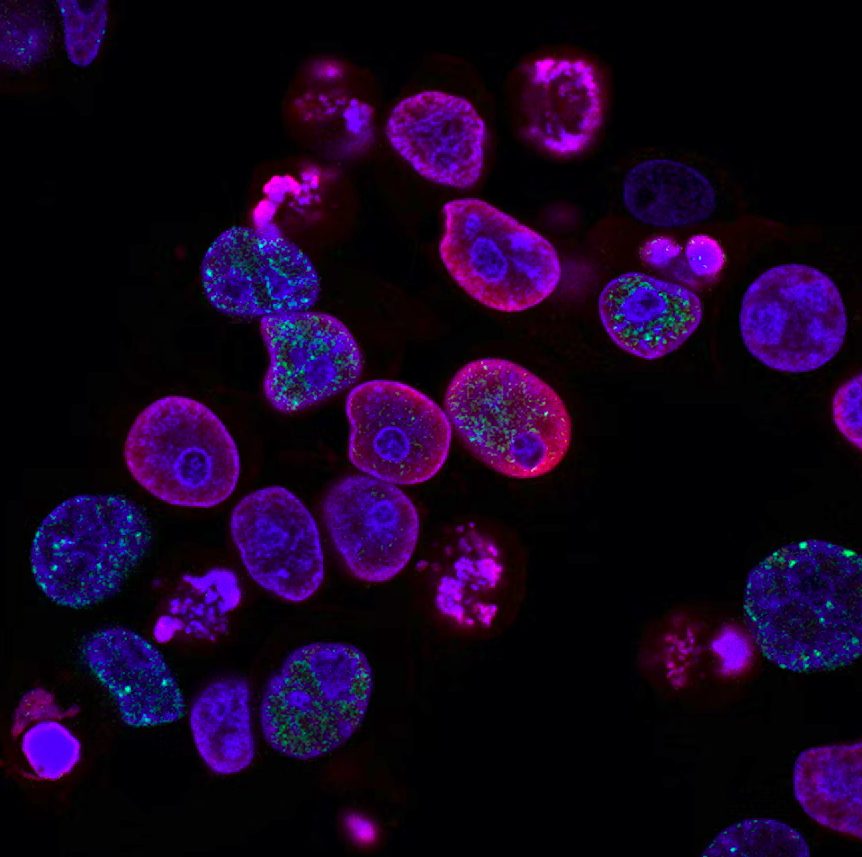
Researchers from Harvard Medical School and National Cheng Kung University have developed a new artificial intelligence model to assist doctors in Colon Cancer treatment.
Colorectal cancer, the second deadliest cancer worldwide, claims 1 million lives every year. This makes it crucial to have a tool that can help save lives by providing accurate and personalized treatment options.
The AI model uses images of tumor samples to accurately predict how aggressive the cancer is. It also predicts the patient’s likelihood of survival with or without disease recurrence. The tool also suggests the optimal therapy for each patient. This provides much-needed clarity to doctors delivering prognoses and making treatment decisions.
With this new tool, clinicians and patients can better understand how to navigate this complex disease. This disease often behaves differently even in patients with similar disease profiles.
It is noteworthy that the new AI model is designed to augment human expertise in treating colorectal cancer patients, not replace it.
The model can perform tasks that human pathologists cannot do based on image viewing alone. The tool could be particularly useful in resource-limited areas where advanced pathology and tumor genetic sequencing may not be readily available.
Kun-Hsing Yu, assistant professor of biomedical informatics in the Blavatnik Institute at HMS and co-senior author of the study
A report on this groundbreaking work is published in Nature Communications.
The tool can guide clinicians to follow up more closely, consider more aggressive treatments. In addition, it can recommend clinical trials testing experimental therapies if the patients have worse predicted prognoses based on the tool’s assessment.
Unlike many current AI tools that replicate or optimize human expertise, the new tool detects and interprets visual patterns on microscopy images. These patterns are indiscernible to the human eye.
Rigorous Training and Thorough Testing
The model was trained on data from 2,000 patients with colorectal cancer, gathered from various national patient cohorts that include more than 450,000 participants. During training, the researchers provided information on patients’ age, sex, cancer stage, outcomes, and tumors’ genomic, epigenetic, protein, and metabolic profiles.
The researchers showed the model pathology images of tumor samples and asked it to identify visual markers for tumor types, genetic mutations, epigenetic alterations, disease progression, and patient survival. The model’s performance was then tested by feeding it images of tumor samples that it had not seen before, and comparing its predictions with actual patient outcomes.
The model accurately predicted the patients’ overall survival and the number of cancer-free years they would have. It also predicted how patients would respond to different therapies, based on the presence or absence of specific genetic mutations that affect cancer progression. It could be especially useful in areas with limited resources for advanced pathology and tumor genetic sequencing.
The model will undergo periodic upgrades to ensure that its performance remains up-to-date with emerging science and new data. The researchers emphasized the importance of continuously monitoring the model’s behavior and performance to ensure that it remains accurate and relevant.
Pinpointing characteristic patterns
A new tumor imaging model makes use of recent imaging technology to identify tumor characteristics. These indicators can reveal how aggressive a tumor is and how it might behave in response to a specific treatment. The model can also identify indicators of genetic mutations in the tumor from an image alone. This task usually requires genomic sequencing. The genomic sequencing can be expensive and time-consuming.
The model’s ability to identify genetic mutations from an image alone is useful in resource-limited settings where genomic sequencing may not be available.
Before deploying the model for use in clinics and hospitals, the researchers suggest a prospective, randomized trial to assess the tool’s performance in actual patients over time. The model’s transparent reasoning is a strength that can increase clinicians’ confidence in using AI models. If a clinician using the model asks why it made a prediction, the model can explain its reasoning and the variables it used.
Measuring disease progression and determining ideal treatment
The model demonstrated high accuracy in identifying image characteristics related to differences in survival rates for patients with colorectal cancer. Specifically, it identified three features that were associated with worse outcomes:
1) Greater cell density within a tumor
2) The presence of supportive tissue around tumor cells
3) Interactions of tumor cells with smooth muscle cells
The tool was able to accurately predict which patients would benefit from immune checkpoint inhibitors, a class of cancer treatments. Moreover, the model successfully detected epigenetic changes associated with colorectal cancer. These changes are known to silence genes that suppress tumors, causing the cancers to grow rapidly. The model’s ability to identify these changes marks another way it can inform treatment choice and prognosis.
Another strength of the model is its transparent reasoning. If a clinician using the model asks why it made a given prediction, the tool can explain its reasoning and the variables it used. This feature is important for increasing clinicians’ confidence in the AI models they use, according to the researchers.